Innovation to Impact. See you at the July ESIP Meeting.
Making Data Matter with Mike Daniels
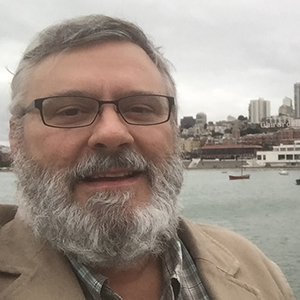
ESIP is 20 years old! To celebrate, we interviewed ESIP community members about their perspectives on the progress of making Earth science data matter from over the last 20+ years. This is the seventh interview to be released. Check out other interviews in the series here.
Interviewee: Mike Daniels, National Center for Atmospheric Research (NCAR)
Interviewer: Arika Virapongse
Date: July 17, 2018
Arika: Could you tell me about when and how you got started working in the field of Earth Science data and informatics?
Mike: I began working with data when I started at NCAR (National Center for Atmospheric Research) in 1981. At the time, attention to data management issues was still fairly new. We were collecting atmospheric data from jet and turboprop aircraft, and using supercomputers with card decks and so forth for processing and quality control of the data. My job was to write code that would plot aircraft time series data in a certain way, which was then used to compare one type of measurement with another. This was my introduction to data handling.
That was almost 40 years ago, and you have been with NCAR this whole time. How has all of this changed over the years?
In those days, all of the processing had to be done on supercomputers. Personal computers came around in the early 80s and in the mid- to late 80s; people transitioned away from using supercomputers for data processing and started using their departmental servers. Shortly after that, UNIX took off at NCAR, and there were a growing number of departmental servers that were UNIX based. So the computing–the processing power and location–moved from centralized supercomputers to PCs and distributed departmental servers.
As another major change, staff were in the field collecting data by carrying out several 8-hour research flights over the period of 1-4 months. The software and data people would send the plane off in the morning. Eight hours later, the plane would come back and the researchers would give them a list of things that did not work mid-flight. In other words, communication with the instrument operators during the flights was virtually nonexistent. Then, in 2002, we took a hand-held Iridium satellite phone and connected it to an antenna mounted on the aircraft fuselage, resulting in our first satellite-based Internet connection to a flying aircraft. This changed everything, because people on the research flight could chat and share data with the people on the ground. If there were instrumentation problems in the air, experts on the ground could help them solve the problem. This led to improved data quality, often saving the flight from being scrubbed. For the first time, we were reliably sending a small stream of data down to the ground, so we could see where the plane was flying and some of the variables that were being measured.
At the same time, new sources of data were growing. We used to send missions out based on limited amounts of data. We would have forecasting models and things like that on paper that we would relay verbally to pilots or scientists via radio. Soon model, satellite, and radar overlays became available, which could be used along with in-situ measurements to guide missions. We could overlay the airborne data on top of the satellite and radar imagery, and then bring all of the sources together into a real-time display to direct the aircraft’s flight track. In-situ sounding data from sondes dropped into storms (e.g. hurricanes) from the aircraft were ingested into forecast models to improve future predictions of a large storm system’s path. So we had a lot more information in real-time about what the current conditions were and where the phenomenon was likely to be moving to next–this was key for improved sampling as the storm changed and developed. The overall objective was to position mobile observational facilities, in the air or on the ground, to optimum locations so that we could intercept and sample the phenomenon being studied at the best point in space and time. It was a very cool to be able to witness this set of breakthroughs.
Which domain areas does NCAR focus on?
NCAR does studies of processes that are not well characterized in models, so we usually research a specific phenomenon. For example, some experiments are related to improving models’ predictions of hurricanes or tornadoes, while others may be focused on chemical exchanges between layers of the atmosphere or within storms as air is mixed and transported. In terms of socioeconomic value, we have historical records that show that old storm track predictions, particularly for hurricanes, varied more widely and were less accurate than today’s predictions. This more data-rich environment has led to better model forecasts of severe storms with lowered uncertainty, which ultimately can save lives through better advanced warnings.
You’ve talked about a progression of how things have improved for collecting aircraft-based data. Can you describe how the political, scientific, and technology context has changed throughout this time to make these changes possible?
Today, our lab at NCAR still gets supplemental funds from NSF (National Science Foundation) for data management and coordination for each large project. So it’s not built in as part of the research project, but rather these services are customized and added on a per project basis. We give NSF and the PIs (principal investigator) the choice for us to provide real-time model, satellite, and radar data– or some of the other background operational datasets that are being created at the same time and in the same place as the experiment. So we give them a menu of options that they can use for their experiments. That menu has now grown in diversity and so has the demand, particularly for things like real-time displays of disparate data. Our staff has also become more adept in how to fuse this data into a display that can be used for situational awareness and mission guidance.
It sounds like the more that you demonstrate the value of what you are producing, then more funds are available for what you need to do. You’ve already mentioned a series of milestones that have happened since you began working in the field of data and informatics. Are there any other events that you’d like to highlight?
Compared to the past, so much more attention is now paid on the quality of the data and metadata. Data stewardship, including data citation, provenance, and tracking, has really improved, resulting in better curated and quality controlled datasets. As we’ve expanded the users and applications of our data, there were times when we recognized that there were some limitations or gaps in our metadata, for example, as new metadata standards or the creation of DOIs (digital object identifier) came into existence. There are also now tools to help with this.
ESIP (Earth Science Information Partners) has played a huge part in this evolution. I am in the NSF world, while NASA (National Aeronautics and Space Administration) and NOAA (National Oceanic and Atmospheric Administration) have been primary sponsors of ESIP for many years. I always come to ESIP thinking: Wow, NSF-funded researchers have fallen behind in many areas. Look at all the advanced features that the other agencies are able to provide–they are using more rich metadata standards that we would love to implement, but don’t have the resources for. ESIP opened my eyes to the work that was being done, and the fact that people shouldn’t be building things on their own but instead be promoting and building collaborative efforts. At ESIP, there are a lot of great people who are willing to volunteer their time, collaborate, talk to you, and help–all for the greater good of Earth Sciences. So the networking aspects of ESIP are just great. I think that ESIP has really pushed forward the general notion of the importance of data stewardship, data citation, and the value of re-using data in ways that we haven’t been able to do within our own agency.
My view is that NSF has a very different culture than NASA and NOAA. NSF focuses more on cutting edge research platforms, with strong participation from the academic community. So we are focused on getting data from newly developed or experimental instruments or research platforms. My experience is that when a new observing platform is proposed, the software, data management, and curation aren’t the first things researchers are typically thinking about. In the case of NOAA, my impression is that stable data services are key for them because they are more operationally focused rather than experimental, so they do a very good job of taking care of and documenting their collections. NASA, for example, might be more focused on validation of what other instruments are seeing relative to the satellites. So this is one of the reasons that they care about in-situ data and comparing them with the remote sensing to verify and validate satellite data. So NSF sits in a very innovative (if somewhat chaotic) nexus of bringing new observational data to a community of academic researchers.
What are some of the major challenges that are facing Earth Science or Earth Science data / informatics today?
The volume and diversity of data. All of the aspects of big data–variety, veracity, volume, velocity–can be challenging. We are at the point where humans can’t deal with these data alone and computers have to help us. But that connection between us and computers–concepts like machine learning and use of intelligent systems to interpret or identify signals in the data–is still pretty new in many areas and requires substantial infrastructure and expertise. The big challenge of today is getting the machines to help analyze the data for reasons needed today but also for what is coming in the future. Due in part to the unique data challenges within disciplines, it can be difficult to get observational scientists and modelers to work together in producing results. In my case, the focus has been on field experiments and handling real time sensors–making sense of and interpreting a network of streaming sensor data with varying spatial and temporal domains to optimize sampling and produce the best quality dataset for scientific analysis.
In addition to what you have already mentioned, are there other solutions that you think need to be developed?
As usual, funding has to be there, especially amongst groups that have traditionally been focused on collecting data but not so much on the metadata, stewardship, and curation of the data. To make these data more usable to machines, you have to have good metadata, follow metadata standards and protocols, and so forth from the very birth of a new platform. Because if you just throw data from thousands of sensors at a computer without making any effort at making them consistent through using controlled vocabularies or interoperable formats, these data are going to be impossible to handle–even for a computer. A layer of consistency among the sources of data is the baseline before you can fully develop the machine-assisted efforts. The funding for technologies to interface data streams to advanced workflows is limited, and the internal structure of the various data providers and consumers can be somewhat stove-piped.
Do you think that metadata standardization is one of those nuts that is impossible to crack?
Groups like ESIP have certainly pushed the concepts forward and there has been lots of talk about the complete reproducibility of data, for example. My own view is that this is the holy grail that might not be 100% achievable. But this doesn’t mean that we shouldn’t work toward it. I can see that full reproducibility is very complicated because one not only has to consult data provider experts who understand the uncertainty in the measurements, but there is also a lot of variation in the workflows used to produce a dataset, such as software versions, customized scripts, operating system differences, format conversions and the like. Just think about the whole environment one creates a research output from–there are so many variables that come into play when a person tries to reproduce someone else’s workflow. We also have to make a judgement on how far to go, because of constraints on resources. If one has to choose, how far does a group emphasize complete metadata versus a well-engineered, stable and documented scientific workflow? It is a balancing act and it seems unlikely that we will get the resources to fully fund reproducibility.
Where do you think that Earth Science data / informatics is going in the future?
That’s a big question, so I’ll just focus on one area. I am working on a real-time sensing project which takes data from small and inexpensive sensors that are becoming ubiquitous in the academic community. We are bringing some standardization to those new data streams through a software tool called CHORDS, which was developed and funded through NSF’s EarthCube initiative. We have shown that the data from one time series platform, such as an aircraft studying the atmosphere, has similar infrastructure and interoperability needs to data coming from a hydrology stream network or from GPS/GNSS (Global Positioning System/Global Navigation Satellite System) sensors measuring the earth’s motion near a volcano. So in terms of sensor streams, there are commonalities in Earth Science data that we can leverage in order to build systems that manage a variety of types of data. In our case, we add sophistication to the sensor streams by providing more standardized ways to access the data across the Earth Sciences, not just within particular disciplines. So in the sensing area, I see many more real-time measurements coming online and these measurements being integrated in a much more consistent way so as to make them more usable to a more broad research community. On the consumer side, these measurements will be connected to more sophisticated science workflows and fed into models to help fill gaps, guide experiments, and improve predictions. At the same time, there will be a lowering of barriers for small groups to provide new measurements to the community across the net, thereby allowing these data to be connected to sophisticated science workflows.
[Disclaimer: The National Center for Atmospheric Research is sponsored by the National Science Foundation. Any opinions or recommendations expressed in this interview are those of the interviewee and do not necessarily reflect the views of NCAR, the National Science Foundation, or any other organizations listed here. This interview also represents an “oral history” (a recollection of history), so its value is in the personal perspectives and insights of the interviewee, rather than specific dates, years, and titles for reference.]