Innovation to Impact. See you at the July ESIP Meeting.
Guest Blog: Bicycle Ridership Modeling in Northwest Arkansas
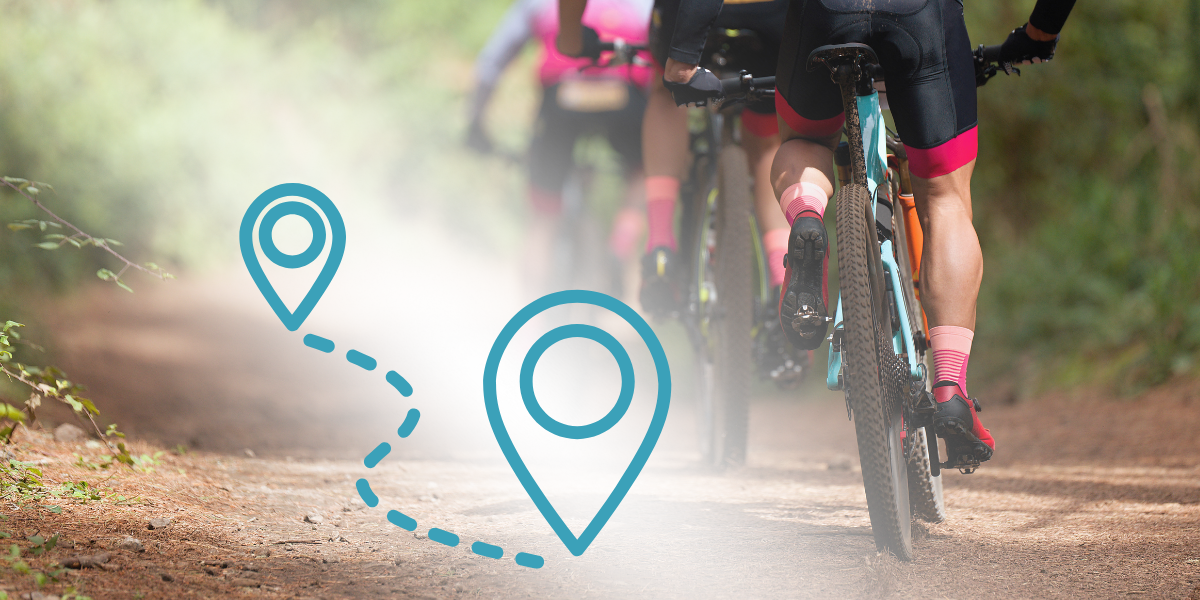
Nelofar Qulizada is an Earth Science Information Partners (ESIP) Community Fellow. In her guest blog, she shares insights on modeling bicycle ridership in northwest Arkansas through her research as a graduate student at the University of Arkansas–Fayetteville.
The United States lags behind many countries in bicycle usage, but there's significant room for growth that could benefit health, society, and the environment. A key challenge for Earth science data experts is combining various data sources – including fitness app information, socioeconomic factors, and environmental data – to create accurate models of bicycle ridership trends. This cross-disciplinary work supports ESIP's goals of enhancing the discoverability, accessibility, and usefulness of Earth science data. The insights could help shape evidence-based policies to promote sustainable transportation and foster more equitable communities.
Even though cycling is the least common mode of travel in the U.S. compared to personal motorized vehicles and public transit, cycling rates have grown in North American cities. As a researcher who has personally experienced the benefits of cycling in NWA, I can see how it can improve the quality of life for people across all demographics, ease the environmental burden by using less motor transport, and build a stronger sense of community through this shared enjoyment of cycling.
Can we lay the path forward for developing a method to count bicycling?
Popular crowdsourced leisure fitness apps (i.e., Strava.com) and other crowdsourced data (i.e., BikeMaps.org) with geographical and socioeconomic variables added in (known as covariate data) can more comprehensively and accurately predict bicycle ridership results within the region. Our role in promoting sustainable transportation is crucial, and this study can provide valuable insights to support those communal efforts.
Many Earth science applications encounter the challenge of combining different types of complex data to derive meaningful insights, whether in climate modeling or ecosystem monitoring. To tackle this, we aim to develop effective data fusion and machine learning techniques to manage these diverse sources' spatial and temporal variability.
What information are we missing?
There is limited research on estimating bicycle volumes, and apps such as Strava tend to attract males between 25 and 35 who use the app for recreational purposes. A general lack of bicycling data, especially bicycling safety data, is prevalent in police reports, insurance reports, and hospital records. The data that is available from these sources is biased towards motorists and only comprises roughly two-tenths of all bicycling incidents, resulting in the trend that traditional sources of information (both in crash incidents and bicycle ridership) underrepresent the numbers of all bicyclists instead of only the user-collected data from fitness apps. The few studies that have measured estimated ridership within an area have calculated the Daily Bicycling Traffic (DBT) or the Average Annual Daily Bicycling Traffic (AADBT) rates categorically (meager to very high) across the study area to model represented results. Bicyclists ‘ safety and perceived safety risks have also reduced ridership rates. Adding bicycle-specific infrastructure to separate motorists and bicyclists has improved these real and perceived risks.
When considering bicycle use in our communities, we run into data problems that Earth science data experts might recognize:
Not Enough Studies: Few studies count the number of bike riders. Like other new research areas, we have yet to get much information.
Biased Samples: Apps like Strava mostly attract young people who ride for fun. This is similar to Earth scientists' struggle to get samples representing everyone.
Missing Reports: Official records like police and hospital reports only capture about 20% of bike incidents. Earth scientists often need help obtaining all the data they need.
Mixing Different Data: We're trying to use data from many places, and each source needs fixing.
These are common challenges in Earth science, too. To deal with these issues, we're:
- Figuring out how many bikes use roads daily and yearly
- Sorting areas by how much bike traffic they have
- Looking at both real and perceived safety risks.
We're doing all this to answer a big question:How do we make good models when we don't have all the data or when our data could be better?
This question comes up in many parts of Earth science, from studying climate to studying ecosystems. By working on these bike use problems, we're not just helping city planning. We're also adding to the more significant talk about handling tricky, real-world data issues in Earth science.
How can we connect all of the pieces to form a complete picture?
The Challenge of Getting Good Data
When we try to get more people to ride bikes, we need to gather and study a lot of information in our area. However, it takes a lot of work to get good data that shows what all types of riders do and experience. This problem is for more than bike studies – many Earth science fields need help getting all the complex information they need.
Working with Limited Information
We want to create ways to make accurate predictions, but we need a lot of good data. Often, this kind of information can be challenging for everyone to access. Earth science experts usually face this problem, too—they must work with incomplete information but still try to make accurate models.
Sharing Our Work and Getting Feedback
We plan to share what we create—our model, the computer code, and how to use it—with other researchers to aim to make Earth science information more accessible. We hope others will try out our work, give us feedback, and help us improve it.
Better accuracy in predicting ridership could help make better decisions about planning cities in ways that are good for the environment. Combining all this gives us a fuller picture of bicycling, which leads to healthier communities, better city design, and less environmental harm.
This blog was written by Nelofar Qulizada with edits from Allison Mills and Megan Orlando.
ESIP stands for Earth Science Information Partners and is a community of partner organizations and volunteers. We work together to meet environmental data challenges and look for opportunities to expand, improve, and innovate across Earth science disciplines. Learn more esipfed.org/get-involved and sign up for the weekly ESIP Update for #EarthScienceData events, funding, webinars and ESIP announcements.