Innovation to Impact. See you at the July ESIP Meeting.
Are Your Data Ready? Take Stock with ESIP’s New AI-Ready Checklist
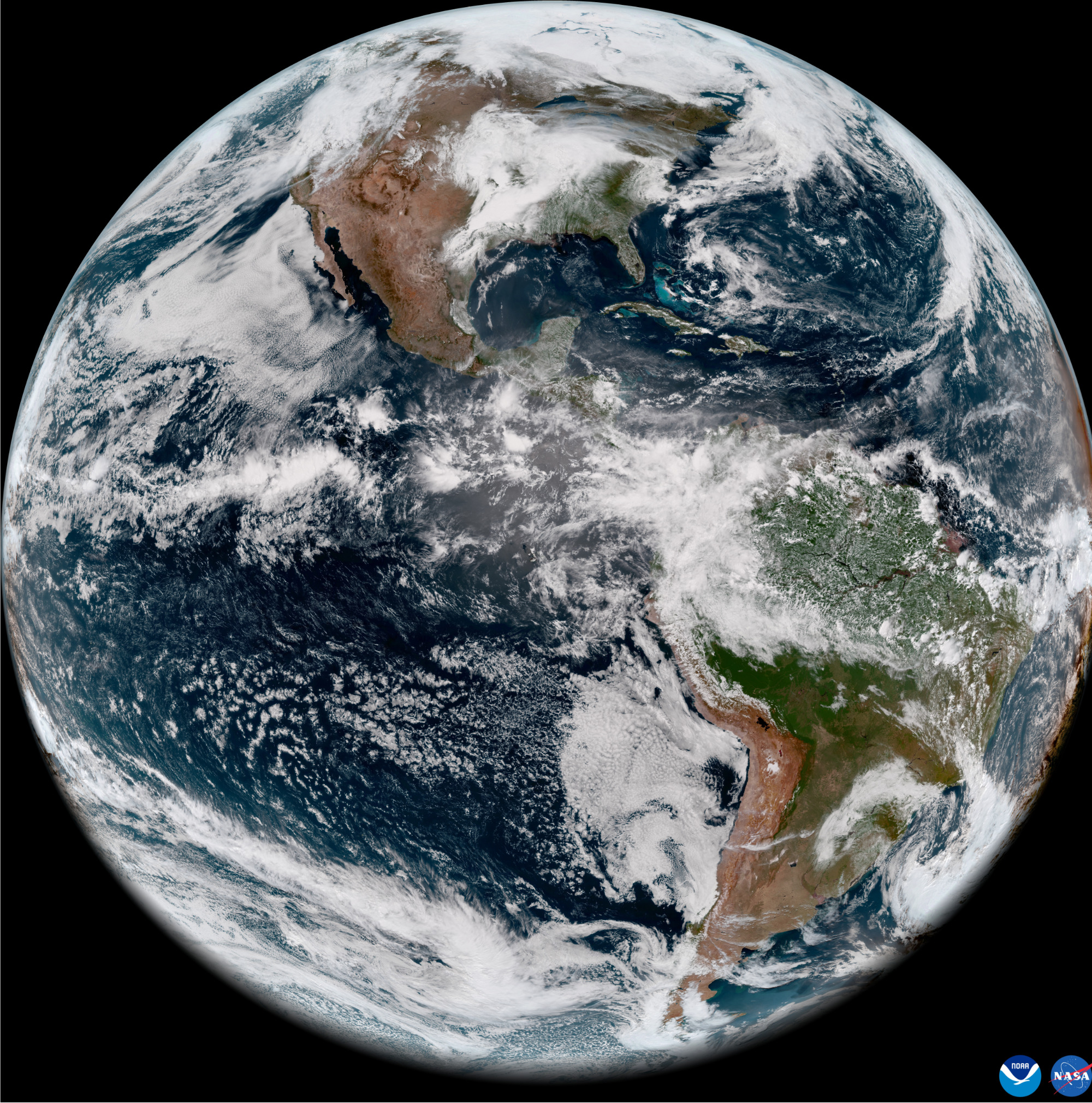
AI-Ready Data
GOES-18 full disk GeoColor image from May 5, 2022. This type of imagery combines data from multiple ABI channels to approximate what the human eye would see from space. Credit: NOAA/NASA
Are your data ready for Earth science applications? The new Checklist to Examine AI-readiness for Open Environmental Datasets can help.
As we aim to develop new standards for data readiness for machine learning (ML) and artificial intelligence (AI) applications, there is an opportunity to improve the usability of Earth science data for our own benefit and the benefit of others.
The Data Readiness Cluster is a group including data managers and scientists who use Earth science data on a regular basis to enhance data analysis and develop new applications through AI and ML. These tools can speed up analysis of bigger datasets, offering an understanding of Earth processes across larger regions and timeframes. For example, ML can help assess regional forest biomass, track changes in snow cover across whole mountain ranges and ice sheets, or analyze decadal trends in hard-to-reach field sites where tundra fires affect one of the world’s biggest carbon sinks.
AI and ML are becoming common and more powerful in addressing Earth science research questions. However, the saying “garbage in, garbage out” still applies to even the most high-resolution, well-intentioned big data analyses. Ensuring that the data are AI-ready means that more research questions can be accurately and efficiently addressed, bias is minimized, datasets can be shareable, reusable and discoverable, and the insights gleaned more closely mirror real-world observations.
Since July 2021, the ESIP Data Readiness Cluster has focused on creating tools like the AI-ready checklist for new data managers and other researchers who are learning to prep data for new uses. The checklist continues to evolve alongside the technologies it supports and its current draft covers key questions needed to ensure data preparation, data quality, data documentation and data access. Cluster participants offer their insights along with explanations of the checklist content.
Our goal is to make environmental data ready for AI application development and reduce the burden of data preparation for users like AI developers.
GOES-18's first images came out in May 2022. The satellite’s Advanced Baseline Imager (ABI) instrument can depict stunning views of Earth. Credit: NOAA/NASA
Data Readiness Cluster
The Data Readiness Cluster is a group of data professionals and AI developers dedicated to developing community guidelines to modernize data management practices for open environmental data.
You can join the Data Readiness Cluster in their monthly ESIP telecon calls on the third Tuesday of each month. Also, catch their Data-A-Thon Workshop using the AI-ready checklist at the 2022 July ESIP Meeting.
Data preparation is the starting point.
No one likes an untidy dataset. Least of all the people who have to go in and clean it up.
Data preparation is notorious for consuming time and sometimes sanity. But it does not have to be: Just as doing the dishes is much easier when you know where to put the clean plates and silverware, data prep is about knowing the right spots to look for problematic data.
The first section of the data checklist focuses on questions that can impede or speed analysis later.
AI-Ready Checklist: Data Preparation
- Have null values/gaps been filled?
- Have outliers been identified?
- Is the data single-source or aggregated from several sources?
- Has the data been gridded (regularized in space and time) or is it in the originally sampled resolution?
- Have targets been identified and labeled (i.e. can this be used as a training dataset for supervised learning techniques)?
We expect data preparation to take 80% of the time for AI projects. AI-ready data helps us move beyond that obstacle, allowing data users to focus on using the data effectively and responsibly while adding value. Of course, what is common is not constant, and the cluster works to agree on common approaches to common tasks that can evolve alongside the technologies.
Data quality is the heart of AI-ready Earth science data.
Through time and space, from size to resolution, from quantifying uncertainty to qualifying review processes. The second section of the checklist takes on questions about data quality.
Perhaps the broadest section of the checklist, there are many resources available. For one, the checklist provides a glossary to define what exactly “completeness” or “integrity” mean in a data science context. Additionally, data quality is an issue that many in the ESIP community have worked on, from establishing global community guidelines for sharing data to making data FAIR (findable, accessible, interoperable, and reusable). The ESIP Data Help Desk galleries are a rich resource of tutorials, one-pagers and links.
Geospatial data reveals a breadth of human experience. Producing beautiful images like this snowy midnight scene from the Suomi NPP Day/Night Band (D/NB) requires data quality, prep, documentation and access. Credit: NOAA/NASA
Having quality-assured data is important to any application. However, it is extremely critical for AI applications as it directly impacts the response of the machine learning algorithms and therefore the validity or relevance of their predictive outcomes.
AI-Ready Checklist: Data Quality
- Have measures been taken to ensure completeness?
- Are there automated processes to monitor consistency?
- Have measures been taken to reduce bias?
- What is the timeliness of the data? (Near real-time,1 week, 1 month, 1 year, more than 1 year)
- Is there a difference between raw near real-time access vs fully quality-controlled data that has an additional delay?
- Are there quantitative measures of uncertainty?
- Is there quantitative information about data resolution in space and time?
- Are there published data quality procedures or reports? (Link to reports)
- Is the provenance tracked and documented?
- Are there checksums / other checks for data integrity?
- How big is the dataset? Depending on the resource, this might be total data volume, dimensionality, number of images, data files, table rows, image size, etc.
- Is this essentially raw data or a derived/processed data product?
- Is this observational data or simulation/model output?
- Has the data been peer-reviewed?
- Has it been down-sampled to reduce resolution, or is it raw? If so, are the raw data available?
Data documentation is about context, use and interoperability.
From finding the data, to knowing how to use them, to respecting the bounds of licenses and privacy, the final sections of the Data Readiness Cluster’s AI-ready checklist offer up questions about how to find and use a dataset.
Documentation is not synonymous with standardization, but the two go hand-in-hand. Returning to our dishes metaphor, well-documented data knows its ice cream bowls from its teacups and that the traditional dinner plate is 10.5 inches across. Data documentation would love cupboard labels and a table of contents, too. While it may sound silly in a household context, there are many steps and categorizations that researchers take for granted in data generation, much like how we all get used to knowing where the pizza cutter goes in our own kitchen. The checklist’s documentation questions offer a map and point to the right people to ask when putting away the data.
AI-Ready Checklist: Data Documentation
- Does the dataset have metadata?
- Is the dataset metadata standardized?
- Is the dataset metadata machine-readable?
- Does it include details on the spatial and temporal extent?
- Is there a comprehensive data dictionary/codebook to describe parameters?
- Is the data dictionary standardized?
- Is the data dictionary machine-readable?
- Do the parameters follow a defined standard?
- Are parameters crosswalked in an ontology or common vocabulary (e.g. NIEM)?
- Does the dataset have a unique persistent identifier,e.g. DOI?
- Is there contact information for subject-matter experts?
- Is there a mechanism for user feedback and suggestions?
- Are there example codes / notebooks / toolkits available showing how the data can be used?
- Is there a clear data license?
- Is the license standardized and machine-readable (e.g. Creative Commons)?
- Has this dataset already been used in AI or ML activities? Link to publications / reports.
- Are there recommendations on the intended use of the data, and uses that are not recommended?
Data is the cornerstone of how the UK Met Office helps people make better decisions to stay safe and thrive, trends in AI have only reinforced this relationship. Data readiness strengthens this vital foundation on which all our data science capabilities are built.
True color satellite imagery gives a sense of the vastness of Earth’s features. To render such images, data has to be carefully documented and analyzed. Credit: NOAA/NASA, Suomi NPP 2016
Good data documentation is the best way to set data users up for success and ensure quality, relevant outputs from your dataset. That’s especially true in the context of high-throughput, automated AI / ML applications.
Data access is the key to being FAIR.
Findable, accessible, interoperable and reusable. FAIR is the open science standard for shareable data.
The principles are not all encompassing – in fact FAIR can be at odds with other frameworks like CARE that emphasize community sovereignty and use. But if AI-ready data as a whole is a dishwasher full of clean dishes ready to get put away, then FAIR helps make sure there is not a lock on the silverware drawer.
Likewise, access can have layers: Knowing the right cupboard is one step, and not having a jumble inside makes it easier to set the table more tidily and efficiently. With this in mind, broader data access invites more users to dinner. Knowing when and where to show up helps diners and data alike, even if some of those users never step foot in the kitchen.
As the final steps of the checklist, data access gets at the whole process’ main goal: AI-ready Earth science data strives to be information that people can use.
AI-Ready Checklist: Data Access
- What is the file format?
- Is it machine-readable?
- Is it available in at least one open, non-proprietary format?
- Is it available in several different file formats?
- Data delivery:
- Direct file download or ordering?
- Is there an API?
- Custom-developed or open, standard protocol?
- For restricted data, have measures been taken to provide some access while still applying appropriate protection for privacy and security?
- Has the data been aggregated to reduce granularity?
- Has the data been anonymized / de-identified?
- Is there secure access to the full dataset for authorized users?
Decision makers must integrate quality Earth science data to meet critical issues. Ultimately every industry and government, including policy makers, utilities and manufacturing, need access to combine usable Earth science data with other domain-specific operational data to foster these informed decisions.
This blog post was co-written by the Data Readiness Cluster participants, Allison Mills from ESIP and Jennifer Fulford from NOAA.
ESIP stands for Earth Science Information Partners and is a community of partner organizations and volunteers. We work together to meet environmental data challenges and look for opportunities to expand, improve, and innovate across Earth science disciplines.
Learn more esipfed.org/get-involved and sign up for the weekly ESIP Update for #EarthScienceData events, funding, webinars and ESIP announcements.